The digital revolution has brought a host of new opportunity and challenges for enterprises in every sector of business. While some organizations have embraced the wave of change and made efforts to be at its forefront, others have held back. These institutions may not have sufficient staff to implement the changes or may be waiting for a proven added-value proposition.
In other words: No technology upgrades until the innovation is proven useful. There is some wisdom in this caution. Several reports have noticed that productivity and efficiency are not rising at expected levels alongside this technology boom. However, as Project Syndicate noted, this lag may be a case of outgrowing the traditional productivity model, meaning that not every employee action is measured in the old system.
However, there is another reason to explain why more technology does not automatically equal greater efficiency and higher profits. If a company buys some new software, it will see a minor boost. However, it will reap the full rewards only if staff properly learn to use said platforms.
Part of this problem stems from the misunderstanding that technology can only improve current work processes. This is not true. When looking at a basic enterprise operation like data visualization, technology has created an entirely new workflow.
Examining the traditional business model
In the traditional business model, all data visualization was manual. Employees would gather data from various endpoints and then input it into a visual model. Common examples of this process included pie charts and bar graphs. The employee would then present the data to the executive, who would use it to make information-based decisions.
While acceptable, this process is far from optimized. Most data had to be generated in spreadsheets before it was collected, using formulas made by staff. Collecting and framing the information is a time-consuming process that will absorb at least one individual. As employees are involved at every step of this workflow, there is a potential for human error.
The time involved prevented companies from acting on real time information. In the interim, intuition and "gut feeling" were used as substitutes for data-backed decisions. The people involved raised the level of risk that the data in question may be inaccurate or misleading.
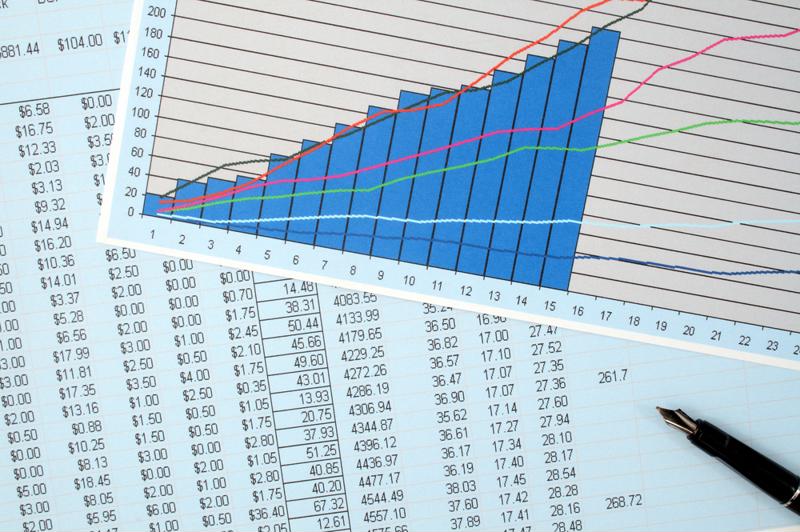
Unlocking data analytics
Of course, with the arrival of the internet of things, companies have a lot more data collection at their disposal. Connected devices have provided a whole new network of information. This gold mine, also known as big data, has one downside: There is too much of it. A human cannot hope to categorize and analyze the information in any acceptable timeframe.
Enter data analytics. Using advanced technology like machine learning, companies can create and implement this software to study their data, creating automatic visualizations based on trends and prevalent patterns. According to Fingent, these software solutions employ mining algorithms to filter out irrelevant information, focusing instead on only what is important.
However, companies cannot simply go from a traditional system to a fully fledged data analytics solution for one reason: data segmentation. Many enterprises divide their information based on different departments and specializations. Each group works internally, communicating primarily with itself. While this method is helpful for organization, it greatly impedes data analytics potential.
If companies have siloed their data, the program will have to reach into every source, work with every relevant software and bypass every network design. In short, it will have to work harder to communicate. While modern data analytics solutions are "smart," they cannot navigate barriers like this easily. They are designed to optimally read only the information that is readily available.
For organizations to fully capitalize on the potential of internal data analytics, infrastructure overhaul is needed. Departments – or at least their data – must be able to freely communicate with one another. This process entails implementing a common software solution that is in use across the entire company.
The good news is that many modern solutions fit this need. Solutions like cloud platforms store relevant data in accessible locations and train employees to not segment their work. By creating an infrastructure that is open to the data analytics program, organizations can start to act on information, rather than relying solely on their gut.
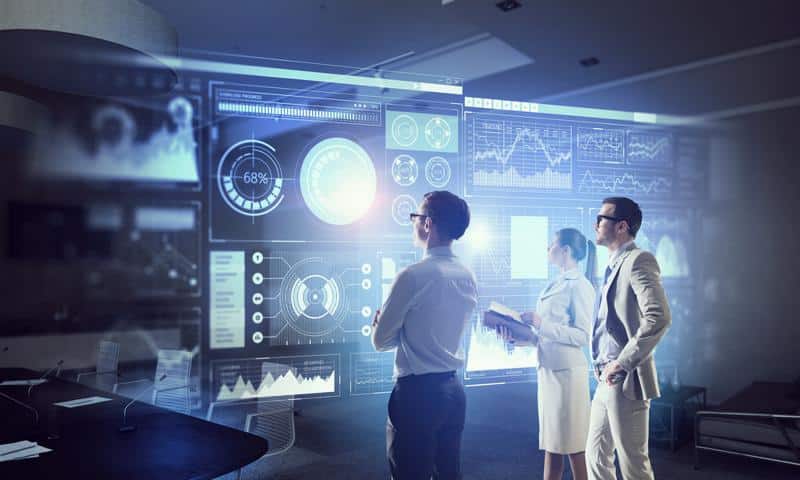